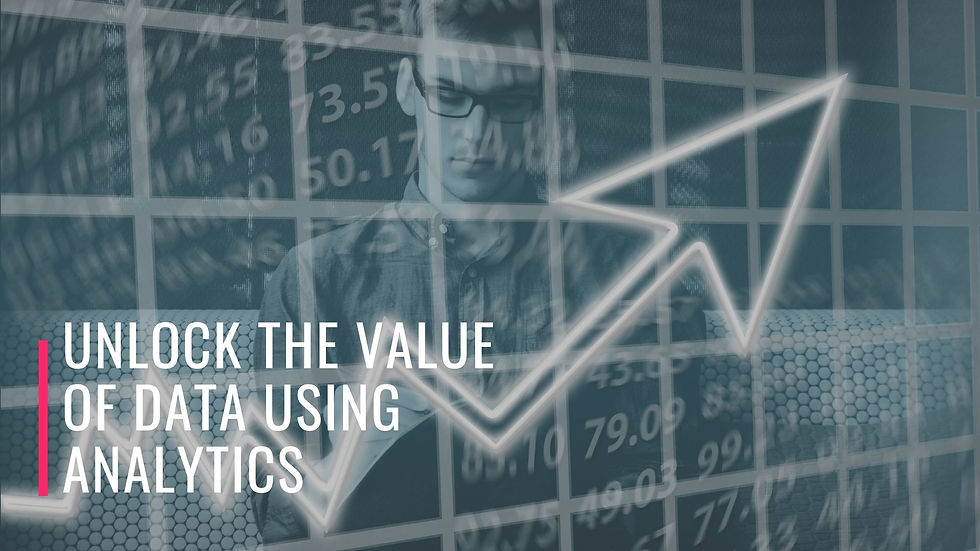
Predictive analytics is a process of using the historical data and different statistical algorithms to identify the likelihood of future events. The predictive analytics has been used for decades now, with various statistical models in use as early as the 80s to predict the stock market.
But only recently, with the rise of competition, growth of volumes of data and the rise of internet, the industry has started understanding the power of predictive analytics. They have made an undeniably strong case for wider adoption of the once unstable technology.
What is Driving Predictive Analytics in Sales?
Growing volumes of data, in part due to more ways to collect them, faster computers for a fraction of the price and cloud computing have all come together to create the perfect environment for predictive analytics.
Even as new technology such as machine learning, artificial intelligence and in-memory computing continue to take root, the impact on the internet economy is expected to be massive. In particular, the latter of these (in-memory computing) has spearheaded the availability of real-time data, greatly increasing the speed with which analytics can be utilized.
In-memory computing is basically the processing of large volumes of data in system memory rather than relying on disk-based processing such as transactional databases. The most impactful implication of this is the resulting speed and performance boost it can achieve.
Few places are as deserving of a complete cultural upturn of mentality as the sales department.
The Impact of Predictive Analytics On Sales
Optimizing marketing campaigns
By feeding a predictive analytics model with historical data, it's possible for the model to come up with information on how your next campaign is likely to perform.
A predictive analytics model can help you come up with data to help you decide which segments you should pay attention to and which parts of the funnel may need improvement.
Actionable insights such as where previous campaigns may have fallen short and what challenges you might face can be derived from the data such models will be useful for enhancing marketing efforts at every stage of the funnel.
Predicting Patterns in User Behavior
The sales funnel was first proposed as a way of simplifying general user behavior into a set of interactions that are easier to work with and since then, it has evolved a great deal. Every customer is unique but they tend to experience the sales process in pretty much the same way.
Along a similar vein, predictive analytics gives businesses an avenue for predicting a visitor's next move. Given a set of data that shows how users interact with your website, it's possible to predict how engaged certain users will be, how and where they drop off from using your product and how likely a customer is to convert.
Using such data, a company can attempt to reach a conclusion regarding why certain users drop off at certain times while others convert, for example. A company may also be able to map certain correlations to each other, such as general location and conversion rates.
Targeted Content Distribution
People are going to experience your sales funnel in what's pretty much the same way. Various factors do differ from person to person, though, such as how many pages they visit, how long they stay and other metrics you probably use to measure engagement. Such data can be crucial in making business decisions.
Using predictive analysis, the kind of blog posts a lead generally reads can be used to inform which kind of content will work best for them. Data such as what kind of device they use the most can also be used to decide whether different platforms are going to be more effective.
You may find that mobile users spend more time in the app than those that visit your site, leading your firm to give more priority to the app.
Dynamic Pricing and Lead Scoring
Most modern companies don't tend to have a fixed pricing model that can be used across the board. Pricing models are made to adapt to factors, such as location, the volume of purchase and customer lifetime value.
Manual dynamic pricing, especially for large firms, is not a viable strategy. A person would have to sit down and analyze potentially hundreds of customers at a time to get anywhere meaningful, and after that, suggest a price based not only on internal factors but external ones like competition as well.
The easiest way is to use collected data to build consumer profiles and models. These models are then scored based on individual factors and grouped according to their value. By doing this, businesses are able to prioritize quality leads that are likely to convert.
Better Segmentation of Consumer Data
Segmentation is the practice of dividing users into different categories based on factors such as age, shopping habits, location and behavior on your site. Segmentation is an essential part of marketing because it helps to smoothen other aspects of data analytics such as personalization of content.
It can be argued that predictive analysis isn't necessary for this since there are dozens of companies out there that don't use it and seem to be fairing on okay. It's also possible to argue for using a spoon to remove screwdrivers from plywood or a knife to chop wood.
While perfect possible to find a knife slim and sturdy enough or a spoon that's shaped just the right way, barring complete unavailability, why not use the right tools for the job?
Granted, segmentation wasn't invented thanks to the sudden availability of data, and traditional segmentation relies on historical data in the same way predictive analytics does. However, traditional segmentation tends to invest more time on old knowledge and not so much up-to-date information. The result is that the sales process becomes less effective - stuck in the past.
By relying on predictive analytics, or more precisely, the data that underlies it, users can be divided into different groups much more accurately. This then enables precise marketing messages based on demographics or even location to be sent to potential customers.
The data can also help to narrow down on the most profitable groups of people and most valuable segments.
Comentarios